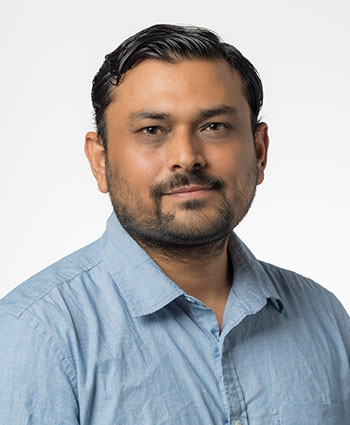
Rimple Sandhu
Researcher III-Computational Science
Rimple.Sandhu@nrel.gov
303-275-4204
https://orcid.org/0000-0003-4415-7694
Rimple joined NREL's wind energy science group as a postdoctoral researcher in 2020. His research focuses on applying modern machine learning and uncertainty quantification (UQ) tools to advance sustainable renewable-energy development. Prior to joining NREL, he was pursuing his doctoral research where he focused on designing efficient Bayesian algorithms using high-performance computing to execute probabilistic modeling of real-life engineering systems. In particular, he was extensively involved in advancing and implementing UQ algorithms such as Bayesian model updating, sensitivity analysis, Markov Chain Monte Carlo sampling, Kalman filtering, Sparse learning, and Bayesian model comparison.
Research Interests
Uncertainty quantification
Bayesian methods
Stochastic simulation
Nonlinear filtering
Computational mechanics
Fluid-structure interactions
Education
Ph.D., Civil Engineering, Carleton University, Canada
M.S., Civil Engineering, Carleton University, Canada
B.S., Civil Engineering, Indian Institute of Technology Bombay, India
Featured Work
Bayesian Model Selection Using Automatic Relevance Determination for Nonlinear Dynamical Systems, Computer Methods in Applied Mechanics and Engineering (2017)
Bayesian Inference of Nonlinear Unsteady Aerodynamics From Aeroelastic Limit Cycle Oscillations, Journal of Computational Physics (2016)
Bayesian Model Selection for Nonlinear Aeroelastic Systems Using Wind-Tunnel Data, Computer Methods in Applied Mechanics and Engineering (2014)
Awards and Honors
Senate medal for outstanding academic achievement, Ph.D. (2020)
Share