Q&A with Andrey Bernstein: Between the Frontiers of Mathematics and the Future of Energy Systems
As NREL’s group manager of Energy Systems Control and Optimization, Andrey Bernstein is busy; not only in volume of research projects, but also in building the future methods of energy system integration: Algorithms developed by Bernstein have become the basis for a growing research agenda named autonomous energy grids.
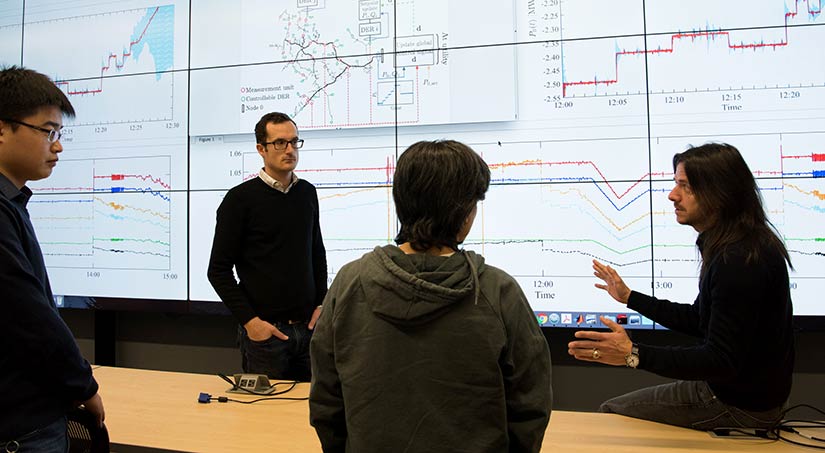
With his expertise in applied math, Bernstein has helped break through the early challenges of real-time optimization and control for distributed energy systems, creating a commercial solution and a community of fellow researchers along the way. Now, Bernstein is revisiting math from his academic past to merge machine learning and distributed control for wide-scale energy systems operation. The following is a conversation with Bernstein, edited for length.
What drew you to NREL, and renewable energy generally?
Let’s say it wasn’t a straightforward route. My background is in applied math, machine learning, and optimization. While finishing my degree in Israel, I started to realize that most of my work was in a bubble—there were not enough applications. At that point, my Ph.D. advisor asked if I wanted to work on smart grid topics in New York City. I hadn’t thought about it before, but the algorithms that I was developing had a very nice application in power and energy systems. I felt excited that it could be applied to important real-world problems. Later, while working on real-time control of power systems in Switzerland, I learned that Emiliano [Dall’Anesee, now assistant professor at University of Colorado-Boulder] and myself were doing very similar work in parallel. When I joined NREL, it was a great opportunity to collaborate on this topic.
How did your research in real-time grid control evolve at NREL?
I was more focused on hierarchical algorithms; Emiliano was more focused on distributed algorithms. Since coming to NREL, I realized that the truth is somewhere in the middle—a hierarchical-distributed approach is very practical, because there are advantages and disadvantages to each. If the system is completely distributed, you need to manage communications between everyone. If the system is completely hierarchical, you need a central authority to control everything. There’s a sweet spot in between. In some sense, we already have a natural hierarchical-distributed structure in power systems. The energy system is embedded within a state, on-top of a city, on-top of a neighborhood.
How are your hierarchical-distributed algorithms applied on the grid?
Currently, our basic structure for small systems is “gather and broadcast,” which is the basis for OptGrid. It means we use central points that gather information, compute some signals, and broadcast those signals down to local devices. In Basalt Vista, for example, controllers at the neighborhood level gather signals from homes and broadcast information back to neighborhood devices. Our approach basically synchronizes the signals, like a conductor that broadcasts coordination to individual players to play their own pieces. This is our basic structure for one cell in autonomous energy systems. Then the analogy gets a bit more complicated, with many orchestras playing…
In the context of autonomous energy systems, how do your algorithms work across multiple domains?
The short answer is that multiple energy systems will eventually work together. Buildings are one asset on the grid, for example, that we want to optimize for itself. Meanwhile, if I optimize for the larger grid, I will see buildings plainly as a power source or power consumer. The two have separate but similar optimizations. It’s a multi-level process, and we want to do these optimizations jointly.
One innovation in our approach with autonomous energy systems is that we are now studying reinforcement learning for energy system optimization. The idea is to have smart decision-making in the face of uncertainty, like “What are the preferences of building occupants?” or “What would the solar production be in five hours?” Reinforcement learning uses trial and error, and continued interaction with the system, to eventually learn an optimal control strategy in such uncertain environments. But we don’t have the luxury of trial and error—we won’t make people suffer in a hot building, or crash a car, just for the system to learn. So, the tricky part is how to balance learning and constraints that make the system work. The good thing in machine learning-based algorithms is that we can apply them in diverse domains, with less difficulty in producing the complex details that concern each system. This is exciting work for me; reinforcement learning methods were also the subject of my master’s and Ph.D. work.
If you were not in renewable energy, which field would you be in?
I have some mathematical passion about real-time decision-making, as well as quite a lot of interest in transportation systems. Autonomy, optimality, and balance of public and private decision-making in the transportation domain is exciting. I’m also interested in weather prediction and the effort to create forecasting methods for uncertain processes. It’s cross-cutting, and I appreciate learning about weather processes. I like nature in general, and I look for any opportunity to get somewhere where I can enjoy and understand nature.
Last Updated May 28, 2025