Digital-Twin Project Green-Lights Traffic Congestion Improvements
Advanced Computing Methods Lead to Reduced Emissions and Shorter Commutes in Metropolitan Tennessee City
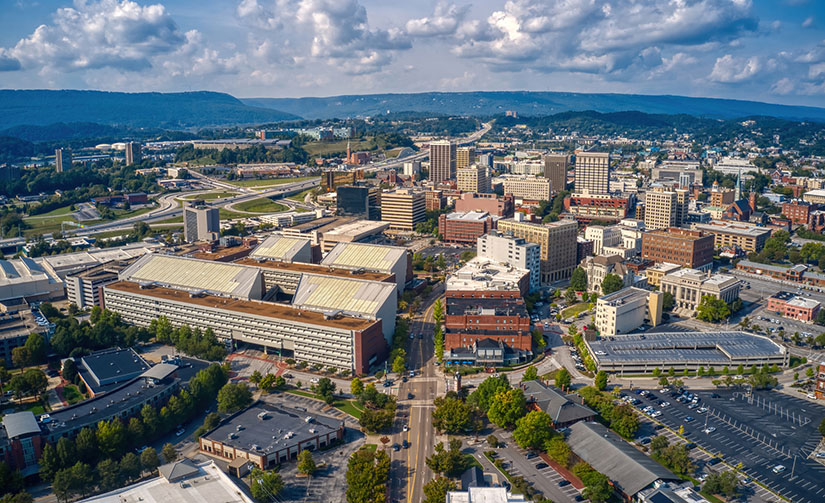
Drivers in Chattanooga, Tennessee, are experiencing smoother commutes thanks to the Chattanooga Digital Twin Project that wrapped up at the end of this past year. The four-year project, a collaboration between the U.S. Department of Energy’s (DOE’s) National Renewable Energy Laboratory (NREL) and Oak Ridge National Laboratory, which led the initiative, aimed to reduce transportation-based energy emissions and commuting delays in the city using advanced computing.
The project, funded through DOE’s Vehicle Technologies Office, has resulted in numerous advancements, including the creation of an effective, transferrable traffic mitigation framework that is capable of powering efforts to reduce traffic congestion nationwide.
Driving Local Solutions
The transportation sector accounts for 27% of greenhouse gas emissions, with the majority of those emissions stemming from light-duty vehicles, or passenger cars. Traffic congestion plays a role in increasing these emissions through stop-and-go fluctuations within traffic patterns and by longer vehicle idling due to traffic-light delays.
To combat these growing emissions, researchers used machine learning and real-time data collected from a wide range of infrastructure sensors to create a “digital twin” depicting Chattanooga traffic conditions. Via sensors installed on roads, this dynamic, data-informed simulation captured real-time traffic conditions, providing researchers with a portal to understand some of the underlying causes of existing traffic congestion. These insights generated from the digital twin allowed researchers to pinpoint specific areas for improvement, test possible solutions, and recommend effective traffic management strategies for city officials.
“Improvements to the transportation network are challenged by both the local topography and the demands of two interstate highway systems carrying over 250,000 vehicles per day,” said Cindy Shell, traffic signal design specialist for the city of Chattanooga. “Improvements like bypasses or ring roads are prohibitively expensive, so we turned to the digital twin project as a cost-effective way to improve the efficiency and resiliency of the existing roadway network.”
In an early phase of the project, researchers ran an algorithm on existing traffic data to determine the optimal timing of signals. Implementing these optimized timings for select traffic signals in Chattanooga resulted in up to 16% energy reduction. Because of the improved traffic conditions, the new recommended timings are still in use by the city of Chattanooga.
Along with optimizing traffic conditions using existing data, researchers used the digital twin capabilities to collect and respond to real-time data. Unlike traditional signal controls that are based on a static schedule, real-time adjusted controls are updated by an advanced computing algorithm in the cloud every five minutes to adjust to prevailing traffic conditions. Responding to conditions instantaneously allows controls to be tailored to optimize mobility. In a real-world deployment in June 2021, these high-performance computing strategies resulted in up to 32% reduction in traffic delays.
Further studies only strengthened the impact that real-time signal control adjustments can have on improving traffic congestion on a larger scale. In a simulation of 39 traffic signals, researchers found these types of synchronously adjusted signals can reduce up to 50% of delays, compared with traditional time-of-day timings.
“This research shows the power of a digital twin to help us fully understand a system, the improvements of developed optimal control algorithms, and the potential impact of artificial intelligence trained in a digital world,” said NREL’s Wesley Jones, a principal computational scientist and co-lead of the laboratory’s project team. “This allows us to avoid disruptions we might have faced if trying to create these solutions in the physical world alone.”
Building a Framework for Future Projects
Along with garnering positive local results in Chattanooga, a pivotal achievement of the project was the development of a transportation congestion analysis and mitigation framework that could be adapted for future use in a variety of contexts.
"The transferable framework we built can acquire the instant traffic data from both the roadway infrastructure and vehicles, make sense of these data, feed the information into the digital twin that we developed to advise the best control decisions, and implement these changes in the real world,” said NREL’s Qichao Wang, computational scientist and traffic control efforts lead for the project.
Part of this framework was the development of a new module that updated a widely used open-source traffic simulation package to include the type of traffic signals used in North America. This important contribution made traffic simulation more accessible to transportation researchers and practitioners in the United States and enabled the success of several transportation-based research projects nationwide.
Along with the cloud-based controls framework, researchers developed innovative methodologies, including a novel approach to measuring excess energy expenditure. These Automated Traffic Signal Performance Measures (ATSPMs) were used to determine which traffic controls to target first to reduce energy expenditure. These measures are now being shared with industry partners, such as the Eastern Transportation Coalition, to provide a tool for local traffic engineers to fully realize the impact of signal timing on excess energy expenditure in their regions.
Another important factor in making these digital twin capabilities operational in various contexts involved recognizing that not all regions have the sensors needed to collect real-time data, as they may be expensive to install and maintain. To solve this issue, researchers were able to use connected vehicle data purchased from data companies to complement existing data sets and provide a more accurate and complete picture when running the simulations.
“Every region has a different set of tools and data available,” said Juliette Ugirumurera, NREL computational scientist and co-lead of the laboratory’s project team. “What makes this project so significant is that we created flexibility within our system that can adjust to the differences across every individual city’s resources and still produce actionable insights that lead to tangible traffic improvements.”
Fueling the Future of Digital Twin Analysis
The development of these capabilities also unlocks the opportunity for diverse traffic congestion analyses, such as examining freight-based traffic congestion. Truck congestion amounted to about $11.3 billion in wasted time and fuel in 2020, making it a ripe opportunity to cut both emissions and costs.
During the course of the Chattanooga Digital Twin Project, researchers developed a machine learning-based methodology to use legacy highway cameras to differentiate between freight and regular traffic, allowing them to understand the volume and movement of freight traffic. In the future, researchers plan to use this data to run simulations and experiments to improve the operational efficiency of truck fleets by optimizing routing.
“This project made a profound impact, both in the positive effect it had on the community and in paving the way for digital twin traffic analyses nationwide,” said NREL’s Vehicle Technologies Laboratory Program Manager John Farrell. “We look forward to seeing the progress and innovation that will come from future transportation partnerships.”
Learn more about NREL’s computational science and transportation and mobility research. And sign up for NREL's quarterly transportation and mobility research newsletter, Sustainable Mobility Matters, to stay current on the latest news.
Last Updated May 28, 2025