Data-Driven Stability Assessment and Control
NREL develops data-driven stability assessment and control approaches to ensure the reliable operation of power systems with high renewable energy penetrations.
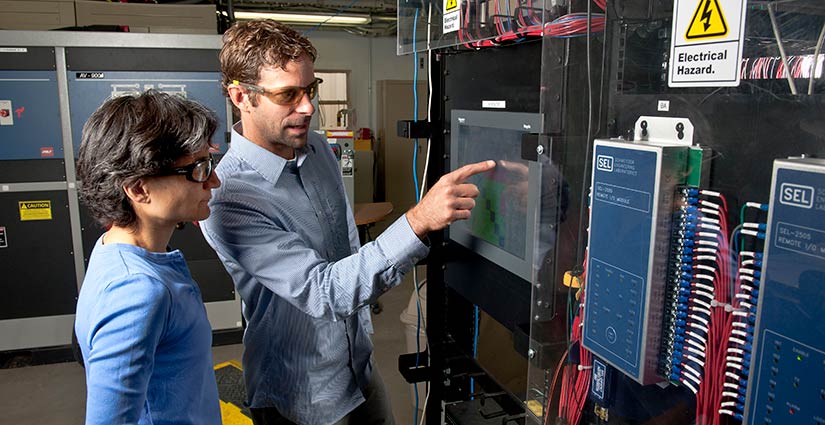
The proliferation of inverter-based resources brings stability challenges, such as low inertia, to power system operations. Traditional model-based stability assessment and control, which require significant computation resources and modeling efforts, will fall short of the fast-changing operation scenarios of power systems with high renewable energy penetrations. NREL research is tackling this challenge from the data-driven angle to explore the potential of massive sensing and measuring data through innovative machine-learning technologies.
Capabilities
- Application of machine-learning technologies to power system dynamic stability assessment
- Data-driven assessment of frequency and rotor angle (transient/small signal) stability
- Data-driven, nonlinear prediction and control to improve stability margin
Publications
On Analytical Construction of Observable Functions in Extended Dynamic Mode Decomposition for Nonlinear Estimation and Prediction, IEEE Control Systems Letters (2021)
Machine Learning-Based PV Reserve Determination Strategy for Frequency Control on the WECC System, IEEE Power and Energy Society Innovative Smart Grid Technologies Conference (2020)
A Review of Artificial Intelligence for Grid Stability Assessment, IEEE International Conference on Communications, Control, and Computing Technologies for Smart Grids (2020)
Data-Driven Participation Factors for Nonlinear Systems Based on Koopman Mode Decomposition, IEEE Control Systems Letters (2019)
Data-Driven Security Assessment of Power Grids Based on Machine Learning Approach, CIGRE Grid of the Future Symposium (2019)
Contact
Share