Dynamic State Estimation
NREL's dynamic state estimation research will enable advanced protection and control schemes that are key to the modernization of electric power grids.
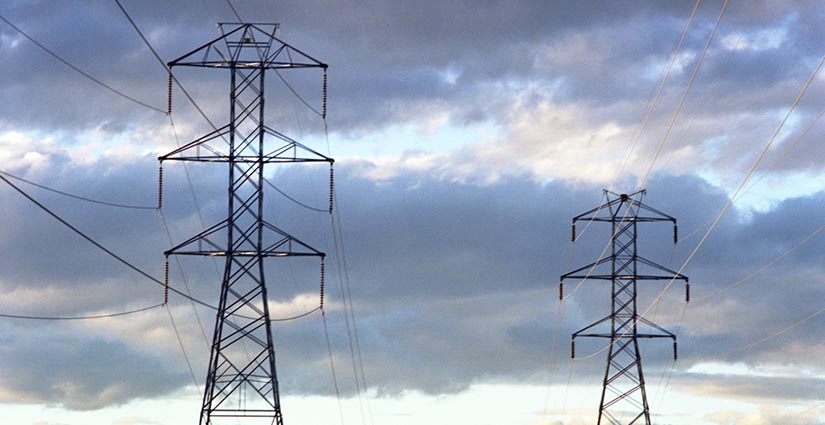
Dynamic state variables carry rich information about underlying system dynamics. Access to dynamic state variables is invaluable for real-time dynamic stability assessments and for designing advanced control schemes. Yet, in practice, dynamic state variables are not measured or even directly measurable.
The alternative is to estimate dynamic state variables based on high-resolution measurements of other variables. Recent developments demonstrate that it is possible to develop dynamic state estimators that can handle data quality issues and model uncertainties. But the scalability of existing methodologies to large networks and measurement redundancy are challenges to be addressed.
Capabilities
- Robust dynamic state estimation
- Centralized or decentralized dynamic state estimation
- Dynamic state estimation based on extended or unscented Kalman filters
- Model-based dynamic state estimators or hybrid dynamic state estimators combining model-based and data-driven methods
Robust Data-Driven Framework for System Identification, Dynamic State Estimation, and Autonomous Control of Electric Power Systems Using the Koopman Operator Theory
This project is based on two pillars. The first pillar is the Koopman operator theory, which allows for the study of nonlinear dynamical systems directly from measured data without relying on a system model. Further, the Koopman operator-based formalism can capture the full nonlinear dynamics embedded in the measured data. These properties are of fundamental importance for future energy grids with high penetrations of variable renewables and power electronics-based converters, which are highly nonlinear. The second pillar is the robust statistics theory, which allows for the development of robust dynamic state estimators. The concept of robustness is key because the developed framework must cope with real-world data issues such as communication noises, data dropouts, and cyberattacks.
Publications
Roles of Dynamic State Estimation in Power System Modeling, Monitoring and Operation, IEEE Transactions on Power Systems (2021)
A Hybrid Framework Combining Model-Based and Data-Driven Methods for Hierarchical Decentralized Robust Dynamic State Estimation, IEEE Power and Energy Society General Meeting (2019)
Power System Dynamic State Estimation: Motivations, Definitions, Methodologies, and Future Work, IEEE Transactions on Power Systems (2019)
A Robust Data-Driven Koopman Kalman Filter for Power Systems Dynamic State Estimation, IEEE Transactions on Power Systems (2018)
A Robust Extended Kalman Filter for Power System Dynamic State Estimation Using PMU Measurements, IEEE Power and Energy Society General Meeting (2016)
View all NREL publications about dynamic state estimation.
Contact
Share
Last Updated March 11, 2025