Control Algorithms for Autonomous Energy Systems
NREL's control algorithms for autonomous energy systems (AES) deconstruct large-scale, centralized control and operations into smaller decisions so that central operators are not overwhelmed by data and communications.
This is an efficient and cost-effective approach to controlling a future grid with large numbers of distributed energy resources and reaping the benefits of innovative technologies with variable generation.
To make this a reality, NREL developed cutting-edge control algorithms around key objectives:
- Real-time operation to balance load and generation every second
- Asynchronous data and control for variance in energy resources and delays in communications
- Robustness, including tolerance to faults, outages, and communication failures and recovery from a failed state
- Scalable design to control hundreds of millions of devices.
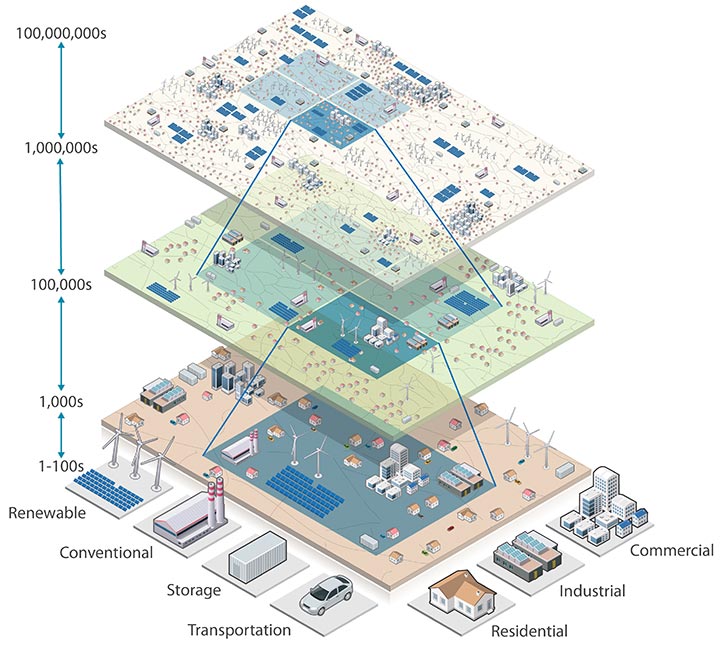
Autonomous energy systems can scale from hundreds to millions of devices.
NREL's AES algorithms automatically connect and disconnect from the grid to operate in grid-connected or island mode, which can result in improved customer reliability, cost reduction, and resilience to grid disturbances. Additionally, researchers developed and tested an algorithmic framework for real-time optimization of distributed energy resources, so that wind turbines or solar PV could provide service to the grid within operational and engineering constraints.
NREL has made fundamental advances in areas such as:
- Multi-area state estimation for distributed communications
- Online distributed optimization for real-time control
- Reinforcement learning for model-free and data-driven optimization
- Consensus-based optimization for distributed decision making.
Workshops and Presentations
Workshop on Resilient Autonomous Energy Systems (2021)
Autonomous Energy Systems: Reimagining Optimization and Control of Future Energy Systems (2021)
Autonomous Optimization and Control of Energy Systems, 14th World Congress of Structural and Multidisciplinary Optimization (2021)
Workshop on Autonomous Energy Systems (2020)
Innovative Optimization and Control Methods for Highly Distributed Autonomous Systems (2019)
Publications
Autonomous Energy Grids: Controlling the Future Grid with Large Amounts of Distributed Energy Resources, IEEE Power and Energy Magazine (2020)
Distributed Minimization of the Power Generation Cost in Prosumer-Based Distribution Networks, American Control Conference (2020)
Good Grids Make Good Neighbors, IEEE Spectrum (2020)
Real-Time Feedback-Based Optimization of Distribution Grids: A Unified Approach, IEEE Transactions on Control of Network Systems (2019)
Online Optimization as a Feedback Controller: Stability and Tracking, IEEE Transactions on Control of Network Systems (2019)
Online Primal-Dual Methods with Measurement Feedback for Time-Varying Convex Optimization, IEEE Transactions on Signal Processing (2019)
Saddle-Flow Dynamics for Distributed Feedback-Based Optimization, IEEE Control Systems Letters (2019)
Wind Direction Estimation Using SCADA Data with Consensus-Based Optimization, Wind Energy Science (2019)
Contact
Andrey Bernstein
Group Manager/Senior Researcher, Energy Systems Control and Optimization
Share
Last Updated March 12, 2025