Machine Learning for Advanced Batteries
NREL uses machine learning (ML)—the next frontier in innovative battery design—to characterize battery performance, lifetime, and safety.
Alongside NREL’s extensive multi-scale modeling, ML can be used to accelerate the understanding of new materials, chemistries, and cell designs. These complex computer algorithms improve battery lifetime predictive modeling and microstructure diagnostics within NREL’s advanced battery research. NREL provides several open data sets to this information and is collaborating with other institutions to standardize battery data.
Machine Learning Increases Battery Life Prediction Accuracy
Reduced-order battery lifetime models use algebraic and differential equations that mimic physical degradation processes. They are interpretable, extrapolate well from small datasets, and are fast to compute. It is difficult, however, to identify accurate model equations. Researchers rarely justify their chosen models versus alternatives in a statistically rigorous manner. To overcome this, NREL applied ML algorithms to automatically generate thousands of equation components and down-select from millions of possible combinations to identify a parsimonious model that balances predictive accuracy with simplicity.
The ML algorithm "rediscovered" relevant physical equations similar to those used by experts but without prior assumptions. Funded by U.S. Department of Energy Vehicle Technologies Office's Energy Storage Testing program, the algorithms are used to diagnose degradation mechanisms, increase life-prediction accuracy, and inform experiment design for the Behind-the-Meter Storage Consortium and eXtreme Fast Charge programs.
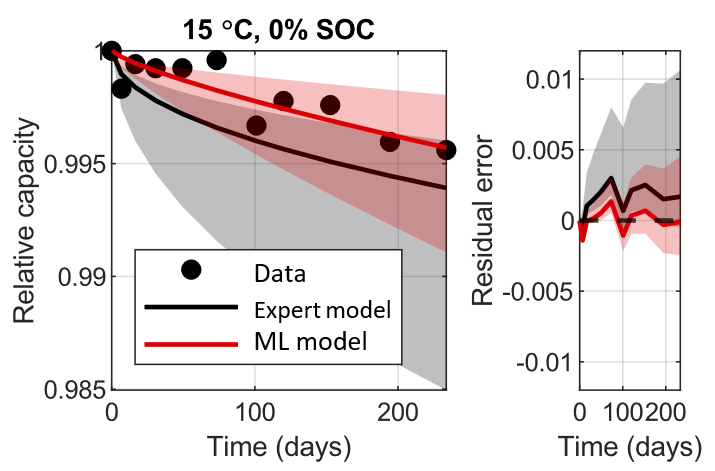
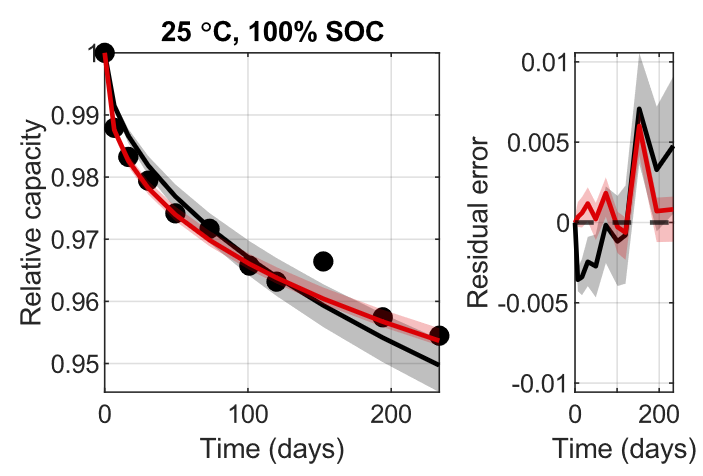
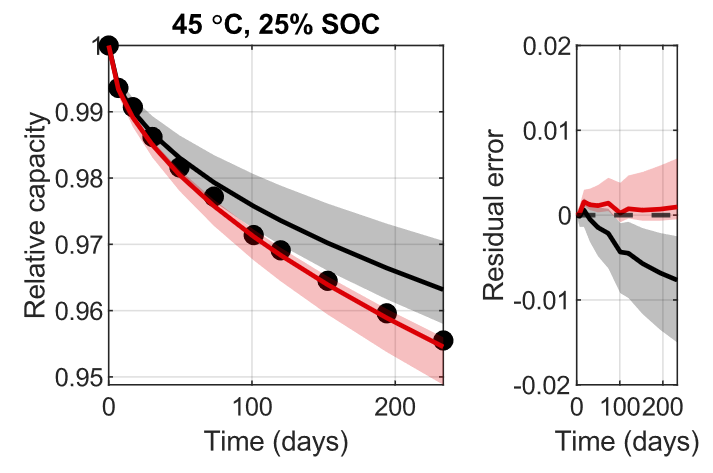
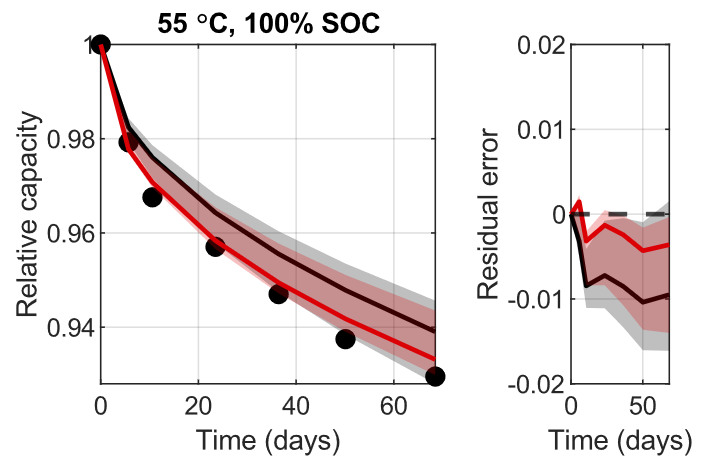
Battery aging data is fit with two models: (i) a literature model based on expert judgment (black with gray 90% confidence interval) and (ii) an ML model (red). The ML model is more accurate on the ~8 months of training data and predicts 40%–130% longer calendar life when extrapolated forward in time, dependent on the aging condition.
Machine Learning Quantitative Microscopy Analysis Informs Multi-Physics Models
Understanding pathways that lithium ions take when intercalating into cathode particles is exceptionally challenging. Polycrystalline features are less than 1 µm in size, requiring resolutions of tens of nanometers. In collaboration with Ulm University, NREL used electron backscatter diffraction (EBSD) to map the orientation and morphology of sub-particle grains in 3D. When combined with ML image segmentation techniques, EBSD provides realistic 3D particle architectures for multi-physics modeling. This combined approach of EBSD, ML, and modeling represents the first demonstration of mapping and simulating dynamic phenomena within single electrode particles.
The ability to determine whether synthesized electrode particles are optimal for performance and lifetime presents manufacturers with an opportunity to measure how well they can control synthesis conditions to achieve favorable particle architectures. Accessible laboratory-based equipment and modeling techniques can inform manufacturers of rate limitations and unfavorable mechanical degradation mechanisms that occur within their particle architectures throughout their lifetime. NREL will next work with other national laboratories to identify optimal electrode particle architectures and guide their synthesis.
Machine Learning Data Processing
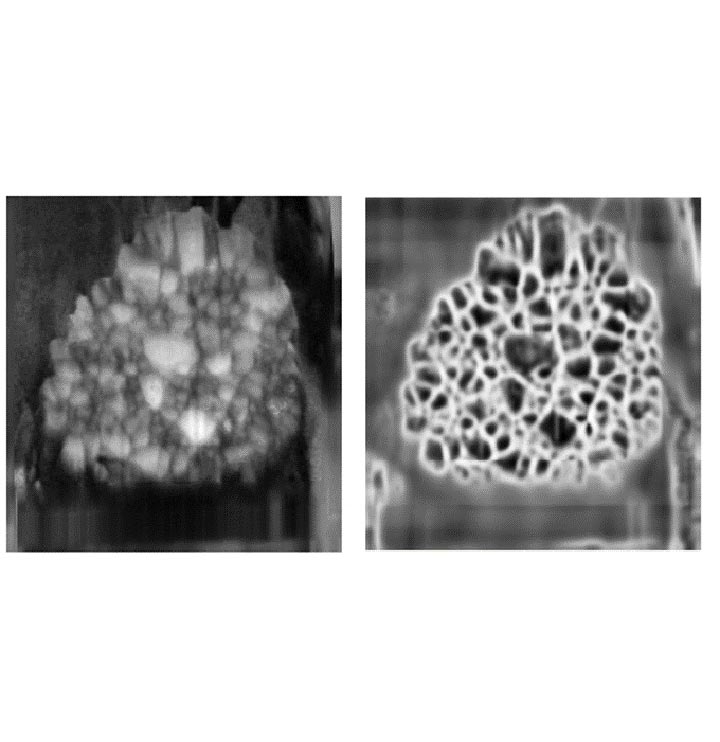
Single Particle Reconstruction
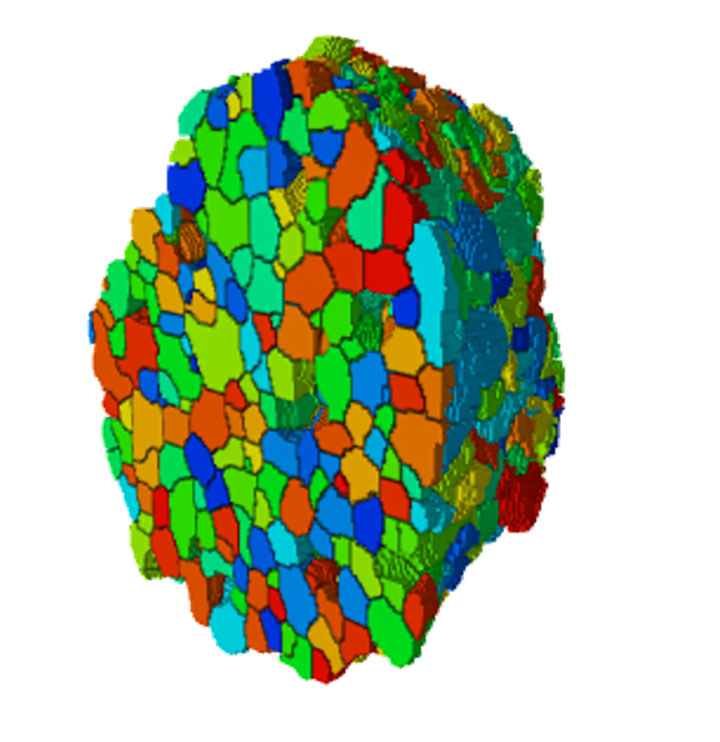
Multi-Physics Modeling
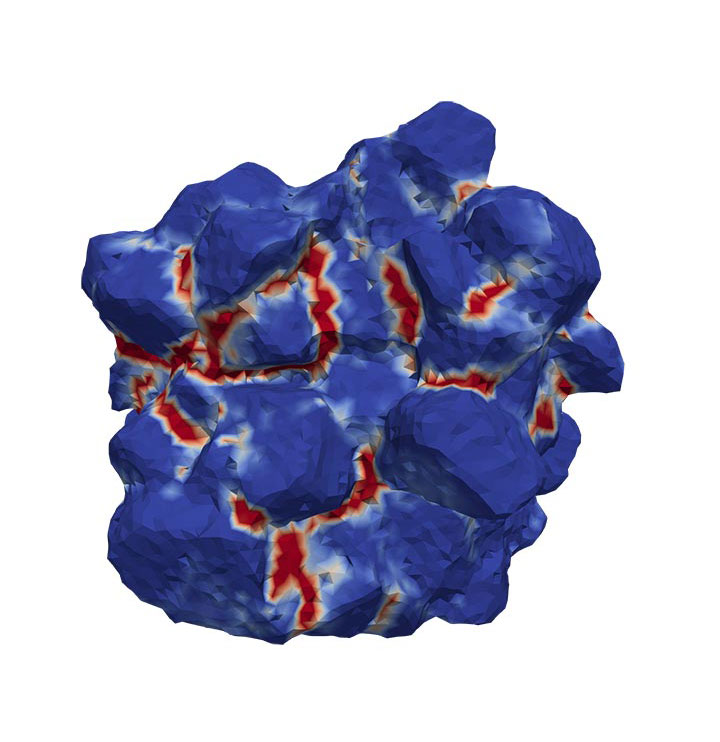
ML data processing facilitates the identification of features within the polycrystalline structure of NMC532 cathode particles, enabling simulation of fast charge damage using multi-physics models.
Open-Source Data
Access to high-quality and robust open-source data is needed to accelerate the development of ML algorithms that match well with complex battery physics. Below are open-source databases provided by NREL for lithium-ion batteries.
Battery Microstructure Library contains X-ray nano-CT data of calendared and uncalendared positive and negative electrodes from lithium-ion batteries.
Battery Failure Databank contains radiography and calorimetry data from several hundred abuse tests conducted on lithium-ion batteries.
Batter Capacity from Electrochemical Impedance Spectroscopy contains hundreds of measurements from commercial cells, recorded at varying temperatures, state-of-charge, and health.
NREL works with other national laboratories and collaborators to release data sets that span the multiple time and length scales of lithium-ion battery applications, providing resources ranging from micro-scale electrode design to grid-scale storage prognostics.
Publications
Machine-Learning Assisted Identification of Accurate Battery Lifetime Models With Uncertainty, The Electrochemical Society (2022)
Predicting Battery Capacity from Impedance at Varying Temperature and State of Charge Using Machine Learning, Cell Reports Physical Science (2022)
Principles of the Battery Data Genome, Joule (2022)
Early Battery Performance Prediction for Mixed Use Charging Profiles Using Hierarchal Machine Learning, Chemistry Europe (2021)
Mapping the Architecture of Single Lithium Ion Electrode Particles in 3D, Using Electron Backscatter Diffraction and Machine Learning Segmentation, Journal of Power Sources (2021)
Identification of Life Models for Li-Ion Batteries Using Penalized Regression and Bilevel Optimization, The Electrochemical Society (2020)
The Application of Data-Driven Methods and Physics-Based Learning for Improving Battery Safety, Joule (2020)
Contact
Share