A Metabolic "Traffic Map": Machine Learning Lets Scientists Plot Complex Flow of Compounds Through Microbes
It Could Help Uncover the Best Routes for Optimizing Microbial-Based Biofuel Production
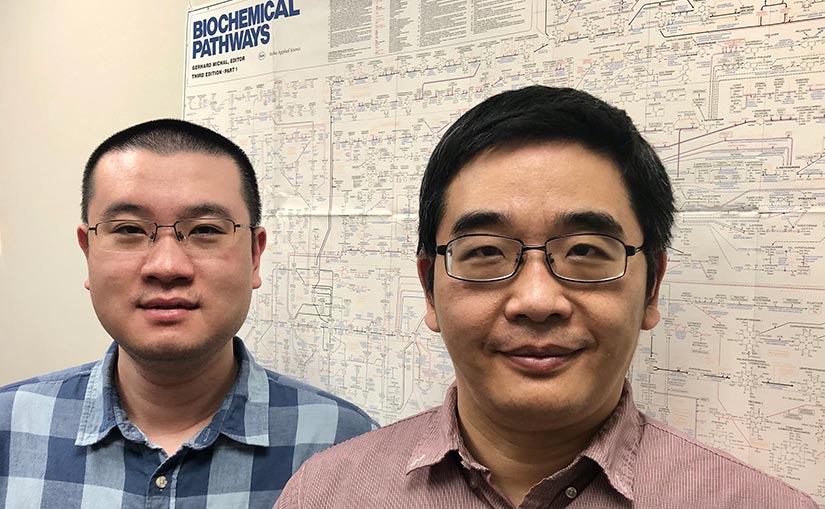
Like traffic signals controlling cars through the center of town, microorganisms use metabolic signals to control the flow of energy and nutrients. Adjusting the timing between a red light and a green light could help ease heavy traffic—increasing the efficiency of a specific biological process. But tweaking a metabolic signal could also lead to traffic jams, bottlenecks, and even gridlock elsewhere in the cell if done haphazardly.
Now, using a new method developed by the National Renewable Energy Laboratory (NREL), scientists could have the information they need to make targeted changes to microbes for system-wide benefits. In an ACS Synthetic Biology paper, "Computational Framework for Machine-Learning-Enabled 13C Fluxomics," NREL scientists Chao Wu, Jianping Yu, Michael Guarnieri, and Wei Xiong describe how machine learning can make it easier and quicker to draft metabolic maps of biological systems.
The work was funded by an NREL Laboratory Directed Research and Development program and the U.S. Department of Energy Office of Science's Biological and Environmental Research program.
"Unlike other machine learning approaches, our algorithm generates data for training and pours them into a computer model to find patterns. It will reduce the model's dependence on experimental data, which are not always sufficiently available or cover all possible scenarios," wrote NREL scientists Chao Wu and Wei Xiong. "This new tool can pave the way for fundamental understanding of microbial systems toward optimal biofuel production."
On Tracking Molecules With Carbon-13

Scientists have long sought to understand how microbes and cellular communities control the flow of metabolites—the energy and nutrients that govern cellular health and productivity. Metabolites comprise fundamental pathways for life, such as glycolysis—the primary route for catabolizing sugar—and the Calvin cycle—the secret of photosynthesis that fixes carbon dioxide in the air to build an organic world. But until recent decades, biologists have struggled to draft reliable maps of this "metabolic flux."
Measuring metabolic flux in biological systems is complex and even daunting—about as hard as counting every car moving through every intersection across the country. In bigger systems, like a human stomach or a fermentation tank full of yeast at an ethanol plant, thousands of metabolic signals can be firing all the time. But why are the signals arranged the way they are? Do cells prioritize some roadways over others? What happens to the flow of traffic if you adjust signal timing?
Previous studies offered methods for uncovering the logic behind these signals. Scientists labeled molecules of interest with carbon-13. As a stable and rare form of carbon, carbon-13 makes it easier to keep track of molecules as they become incorporated into a microbe's network of metabolites and reactions.
But carbon-13 analysis—while still the golden standard of measuring metabolic flux—demands a lot of time and computing power. Mapping traffic in a large biological system was out of the question.
Smart Algorithm Makes for Smarter Bioengineering
NREL's method overcomes that challenge by making carbon-13 analysis more efficient using a smart algorithm that rapidly analyzes data sets from carbon-13 flux experiments. "Essentially, our tool boosts the efficiency of metabolic phenotyping and pathway design in synthetic biology," Wu explained.
Even with limited data, the tool can train itself to rapidly predict metabolic flux, generating a map showing how—and how fast—carbon-13-labeled metabolites progress through the system. The paper details how the approach reduced computation time for metabolic flux estimation in E. coli to less than one second with pretrained models.
On the highway toward transforming biomass into climate-friendly biofuel at a large scale, optimizing the flow of metabolic traffic in microbes could be paramount. Although microbes already excel at digesting or fermenting plant material into compounds needed to make fuel, scientist think they might be engineered to become even more effective.
"Assuming A is a feedstock and B is a target biofuel molecule, we want to know how fast a car can go from A to B—how to design the best traffic system to realize optimal biofuel production," Xiong said. "Our method helps us know where traffic jams happen and, furthermore, how we could control the green and red lights on the 'road' for effective traffic management."
This is part of a series on NREL's bioenergy machine learning research. Read the first story, "Machine Learning Method Could Speed the Search for New Battery Materials."